IMDB is a website that aggregates various pieces of data about movies and television series. Users can submit their own data, including reviews and ratings. IMDB has several datasets available for download, one of which is the rating data for every entry in their database.
I chose to look just at TV series and even within that set of data, there is a lot of interesting information. One note on the data: users can rate an entire show on the show’s main page and/or rate individual episodes. This data only looks at the individual episode ratings.
Best and Worst TV Shows
The top rated and lowest rated series was easy to find, but I wanted to exclude odd, esoteric series that few people have seen. These tables show series that have an average of at least 250 votes per episode.
Best TV Shows:
Series | Avg. Score |
South of Sunset (1993) | 9.400 |
Planet Earth (2006) | 9.155 |
Band of Brothers (2001) | 9.090 |
Anything But Love (1989) | 8.900 |
Fawlty Towers (1975) | 8.867 |
Game of Thrones (2011) | 8.810 |
Breaking Bad (2008) | 8.807 |
Firefly (2002) | 8.764 |
The Wire (2002) | 8.763 |
Freaks and Geeks (1999) | 8.761 |
Worst TV Shows:
Series | Avg. Score |
Ben Hur (2010) | 5.700 |
Beck (1997) | 5.923 |
Wallander (2005) | 5.967 |
Fear Itself (2008) | 5.969 |
Masters of Horror (2005) | 6.1423 |
Veronica Mars (2004) | 6.344 |
Rags to Riches (1987) | 6.400 |
Knight Rider (2008) | 6.656 |
Stand by Your Man (1992) | 6.800 |
The Shield (2002) | 7.000 |
My wife says this can’t be accurate because “Veronica Mars is a great show,” but I guess the internet disagrees.
Another way of controlling what gets placed into the top and bottom is to look at overall votes. By looking at series with a total of at least 1000 votes, we get to see shows that (generally) span a longer time since more episodes equals more votes.
Best TV Shows:
Series | Avg. Rating |
Planet Earth (2006) | 9.155 |
Band of Brothers (2001) | 9.090 |
Fawlty Towers (1975) | 8.867 |
Absolutely Fabulous (1992) | 8.858 |
Saving Grace (2007) | 8.817 |
Game of Thrones (2011) | 8.810 |
Breaking Bad (2008) | 8.807 |
Oz (1997) | 8.771 |
Firefly (2002) | 8.764 |
The Wire (2002) | 8.763 |
Worst TV Shows:
Series | Avg. Score |
House of Payne (2006) | 1.260 |
Renegade (1992) | 2.399 |
The Simple Life (2003) | 2.695 |
MADtv (1995) | 3.807 |
Witchblade (2001) | 4.113 |
Rosamunde Pilcher (1993) | 4.362 |
The Tonight Show with Jay Leno (1992) | 4.385 |
American Idol (2002) | 4.453 |
Dancing with the Stars (2005) | 4.530 |
Baywatch (1989) | 4.782 |
Even with this adjustment, many of the top shows are the same. However, the bottom shows are a bit more interesting in this table. We see shows with many seasons and no story arcs (e.g. talk shows), but also the controversial sitcom House of Payne.
Consistency
Sticking with shows that have a total of at least 1000 votes, I wanted to see which shows are most and least consistent. To find this, I subtracted the minimum episode rating from the maximum episode rating.
Most Consistent Series:
Series | Difference | Avg. Rank |
Planet Earth | 0.1 | 9.15 |
Black Mirror | 0.2 | 7.93 |
Murder Rooms: Mysteries of the Real Sherlock Holmes | 0.3 | 7.88 |
Police Squad! | 0.3 | 8.20 |
Taken | 0.5 | 7.62 |
The Hollow Crown | 0.5 | 8.13 |
Band of Brothers | 0.5 | 9.09 |
Day Break | 0.5 | 7.77 |
Forbrydelsen | 0.6 | 7.73 |
Generation Kill | 0.6 | 8.41 |
Least Consistent Series:
Series | Difference | Avg. Rating |
Jimmy Kimmel Live! | 8.8 | 4.85 |
The Tonight Show with Jay Leno | 8.8 | 4.38 |
ABC Afterschool Specials | 8.6 | 5.82 |
SpongeBob SquarePants | 8.0 | 7.28 |
Disneyland | 7.9 | 7.18 |
Horizon | 7.7 | 7.53 |
Big Time Rush | 7.7 | 4.98 |
Hawaii Five-O (1968) | 7.7 | 6.89 |
Video on Trial | 7.6 | 6.62 |
Screen Two | 7.6 | 6.78 |
Note that consistency doesn’t necessarily correlate with overall rating (even with all shows plotted out). Taken and Sponge Bob have similar overall ratings, but they are very different in terms of consistency.
“I can’t believe they cancelled ____” and “That show is still on?”
I pulled the best shows with only one season and the worst shows with at least five seasons. To be fair, some of the one-season shows are intended to be mini-series. However, you’ll also notice many cult classics.
Top Rated Series with One Season:
Series | Avg. Score |
Planet Earth | 9.155 |
Band of Brothers | 9.090 |
Firefly | 8.764 |
Freaks and Geeks | 8.761 |
Spartacus: Gods of the Arena | 8.667 |
Mr. Bean | 8.579 |
Wonderfalls | 8.450 |
My So-Called Life | 8.432 |
Shin seiki evangerion | 8.423 |
Generation Kill | 8.414 |
The worst shows that have at least five seasons are particularly interesting because it shows a disconnect between the people who rate TV shows on IMDB and the public at large. These shows are or were profitable otherwise the television companies would cancel them.
Worst Rated Series with At Least Five Seasons:
Series | Avg. Score |
House of Payne | 1.26 |
MADtv | 3.81 |
The Tonight Show with Jay Leno | 4.38 |
American Idol: The Search for a Superstar | 4.45 |
Dancing with the Stars | 4.53 |
Baywatch | 4.78 |
Jimmy Kimmel Live! | 4.85 |
Walker, Texas Ranger | 4.91 |
7th Heaven | 5.32 |
Big Brother | 5.33 |
Rating Distribution
In looking at this data, I noticed that it’s pretty hard for a show to get a low rating. The large majority of shows are in the 7-9 range, and very few shows have an average below 5.0. The median rating was 7.4 which may be a side-effect of a 1-10 rating system. Notice also the two up-ticks at 1.0 and 10.0. (Click to enlarge)
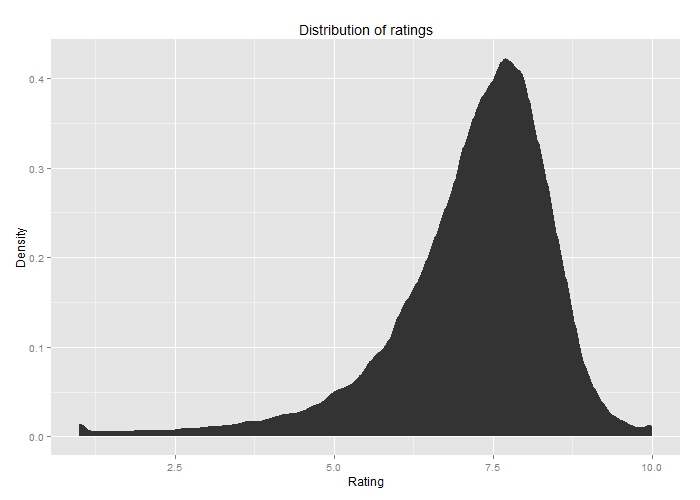
A distribution graph of the ratings of TV shows on IMDB.
I was curious to see if the number of votes changes the distribution. This chart shows the four quartiles of the number of votes per episode. (Click to enlarge)
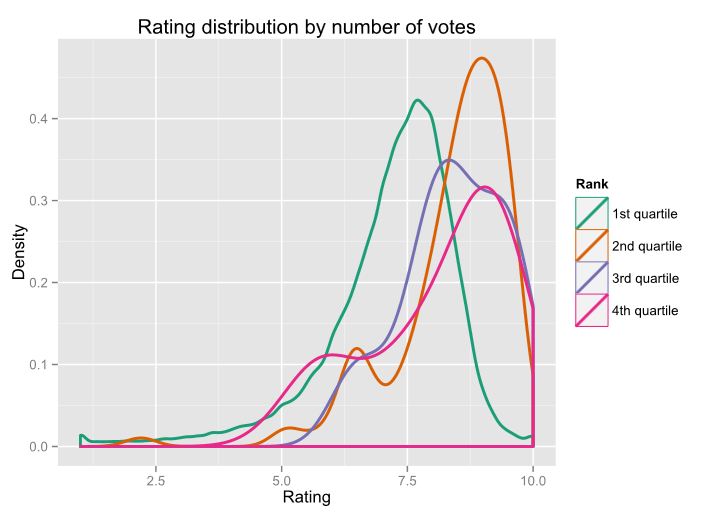
Distribution of IMDB ratings, broken down by quartile.
The first quartile is almost identical. The fourth quartile is interesting because it has two distinct modes. If a popular show has a bad episode, it likely draws in more people to vote giving us the peak around 6.0.
Since the episodes in the fourth quartile are also often the highest-rated, I wondered if there was a correlation between number of votes and rating. Logically, it makes sense that the better a show is, the more popular it is, and therefore more people will be voting on it.
This chart has jitter applied because I found the distinct bars to be distracting. (Click to enlarge)
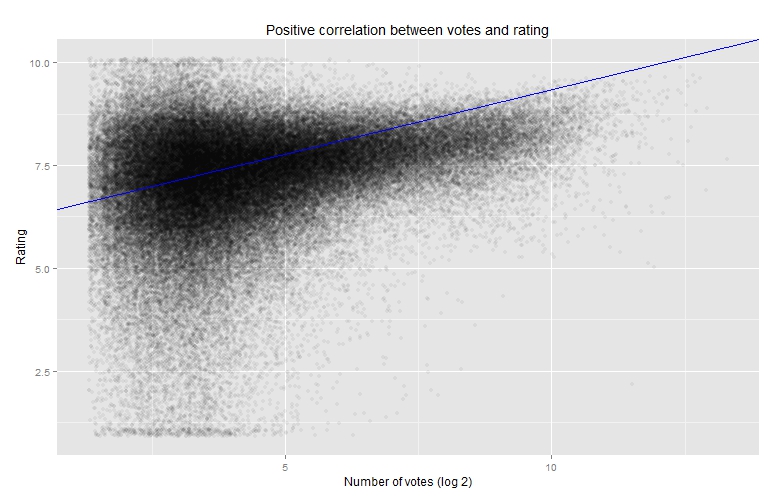
Comparison between the number of votes and avg. score.
The correlation is there, but it is loose (R=0.092).
<h4>Shows over time</h4>
My opinion is that a good show should only rarely go past five seasons. Most of my favorite shows are ones that knew when to stop. This chart shows the distribution of episodes according to season (outliers excluded). (Click to enlarge)
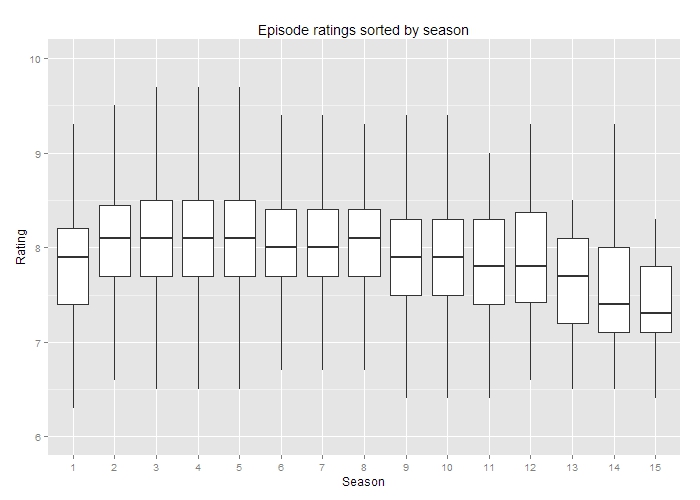
The average TV series rating by season
My five-season marker seems to hold true in this chart. There’s a noticeable dip for sixth and seventh seasons. And after eight seasons, it’s mostly downhill.
<h4>Golden age of TV</h4>
Many critics agree that television in the past few years is the best it has ever been. We’re getting movie-quality writing, acting, and directing over dozens of episodes. To test this, I plotted the median rating per year since 1980. (Note, the data set shows the year in which the series started, not the year of the episode itself.)
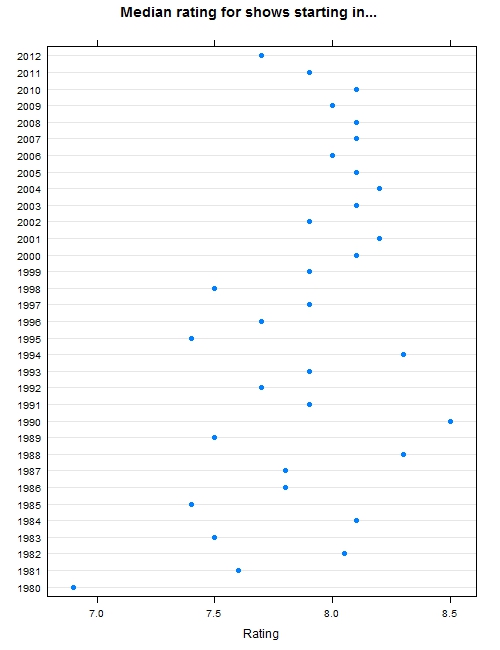
The median TV series rated for each year.
While not definitive, you can definitely see that starting in 2003, TV has been moderately consistent. This is change from the 80s and 90s when it was more hit or miss.